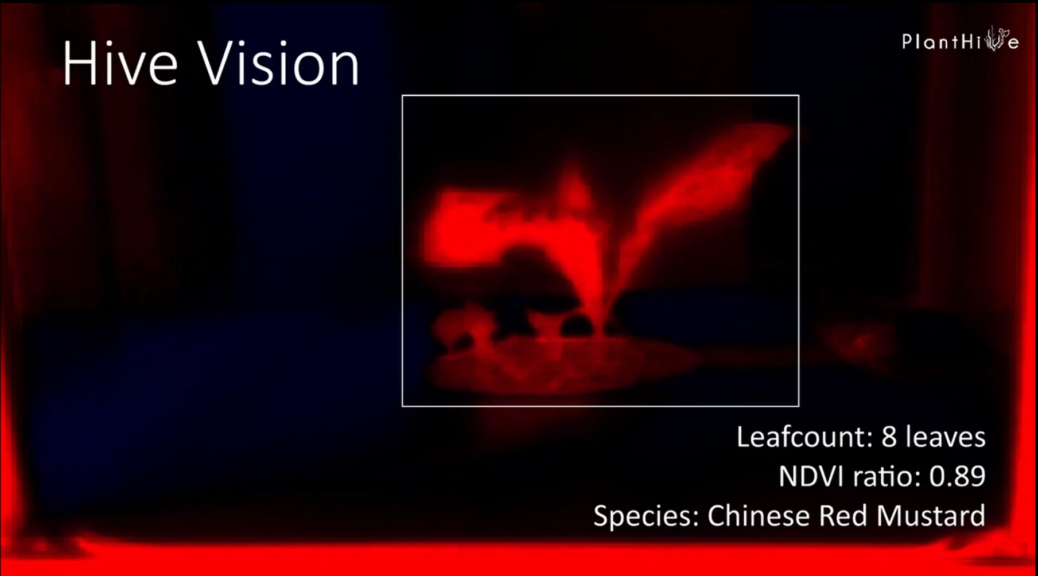
Remote-sensing technology : the Hive-Vision Module!
When deep learning algorithms meet plant agriculture to produce a new domain of application in the ever expanding field of machine learning, we knew that we came across something that couldn’t just be ignore and sweep somewhere in our favorite Sci-Fi video collection. Yes, we are talking about taking a further leap in the merging of electronic printed circuit boards with vegetal multi-cellular life. How? It’s called HiveVision.
The problem of perception
Being primate animals, humans are a highly visual species. So, the most natural thing for our team to do was to equip our PlantHive with a high-resolution camera to get it closer, evolutionary speaking, to our farming hobbyist friends. However, embedding a machine with some kind of multi-array photon detector won’t be sufficient to awaken the eyes of our PlantHive HiveVision camera module. This was an intriguing barrier for AI researchers to overcome and has been named the “problem of machine perception”.
Just try this quick thought experiment. Close your eyes and try to forget that the world is made out of “things”, that can be distinguished by their shapes, color, smell or touch. Forget that those things have names, tags or attributes that can be listed in your neurological dictionary.
Open your eyes, what do you see? Well, if you fell into some kind of meditative trance you’ll be seeing “nothing”. The world would seem like a big void, an infinite space of endless possibilities and interpretations. Wouldn’t it be impossible to “know” what to concentrate at, by looking at this picture below? Would you be able to distinguish between the background and the foreground?
If you flushed your whole repository of shapes and forgot every single tag you have been taught to label a particular pattern, how are you going to be able to extract it out of this infinite landscape of potentially extractable things? What a scary sensation!
As a matter of fact, this is exactly what PlantHive camera would feel like… Kind of… Because cameras have feelings too! Somehow in order to perceive, it has to be told what to see: first by restraining its field of vision to a particular pattern that distinguishes itself from the rest on the basis of its color or shape, and secondly, tag it with a word. By extracting, say the green channel from that picture and plot it on a grey scale, it would look something like this:
Every single green pixel – in this case the leafs of our chili pepper plant – is isolated and it will become just a matter of choosing the right color channel to give a good definition of what constitutes the “background” (black) and the “foreground” (white). Having an additional reference measures, for instance the distance between the camera and the one of the PlantHive columns and the height of the latter, we can compute the surface of the canopy. Furthermore, your HiveVision module is able to take pictures in the near infrared (NIR) spectrum, enabling to assess the reflectance of infrared light from foliage, which amazingly correlates with the concentration of chlorophyll! Just have a look at the GIF below, where blue-red color gradient gives a relative measure of photosynthetic activity. Red corresponds to healthy leafs whereas blue pixels (stem) indicate low chlorophyll concentration.
In the beginning was the word…
Let’s say we want to have a qualitative analysis of our plant, maybe identifying its species. This is where deep learning comes into play. As the name already suggests, HiveVision module is “trained” by taking a large set of images for instance of different pepper plants and “tag” those images with a label, containing information on what the camera is supposed to see. In this way, the camera (more accurately its processing unit) constructs a model of how a pepper plant is supposed to look like and next time it takes a snap of a plant it will be able to tell whether it’s a pepper plant or not, by just analyzing the shape of its leaves and comparing it with an already trained model.
This a priori structure was, as empirical philosophers of the 18th century like Emmanuel Kant had already theorized, necessary and sufficient for a perceptual experience to arise from a merely passive observers submerged in this infinite universe of pixels distributed on a XY axis.
Remote plant diagnostics and remedy
Sensing without contact? Yes we can! Since the Planthive is equipped with a hygrometer, we can correlate the evolution of leaf size and appearance with ambient humidity and temperature. If for example (as shown in the illustration below), leafs are beginning to look depressed, our HiveVision module will be able to link this change with a sudden decrease in humidity and take necessary actions. This includes for example, activating the humidifier to stablize humidity to its normal value and/or readjust light spectrum from blue to red in order to reactivate the production of chlorophyll.
An AI scanning your plants, combined with a smart greenhouse regulating its own environment sounded until now like another Philip K. Dick novel! In this case, the T-X 1000 would have been, probably, not advanced enough babysit your favorite plants. Luckily, your Planthive is taking care of everything!
Don’t Miss any PlantHve news! Stay tuned on our website and blog!
This entry was redacted by our crazy scientist, Federicco Lucchetti, and edited by Vasileios Vallas